AG2PI Field Day #27 - June 21, 2023
Homomorphic Encryption to Enable Sharing of Confidential Data in Agricultural Genome to Phenome
June 21, 2023
10:30 AM - 12:00 PM
(US Central Time)
Purpose
Demonstrate a new method of encrypting data that preserves privacy of proprietary information.
Registration
Register for the virtual event by clicking the link below. Upon registration, you will receive a confirmation email with information about joining the meeting
Field Day RegistrationField Day Recording
Field day recording is now available, click the button below to launch and watch the recording
Watch Field Day Recording GitHub RepositoryData sharing is essential for agricultural research and many funding agencies and journals now require research data to be made public following the FAIR (Findable, Accessible, Interoperable and Reusable) principles. However, concerns remain in keeping confidential and sensitive information protected. Secure data encryption can acheive this while still allowing the data to be used for validation and further research. Among secure encryption methods, homomorphic encryption (HE) refers to encryption that obscures confidential aspects of the data without affecting the outcomes of certain computations. Drs. Cheng and Dekkers will present an HE method called HE of Genotypes and Phenotypes (HEGP). This method is based on high-dimensional random orthogonal transformations. We will show that HEGP accommodates most widely-used mixed models for genomic prediction, GWAS, and genetic parameter estimation, including Bayesian variable selection methods. We will also show that HEGP enables the combining of data for joint analysis when each data set is encrypted with its own key. This allows each contributor to use its own private key prior to sharing the data, without having to share the key.
In addition, Dr. Steibel will present his AG2PI funded work on models that enable multi-institutional collaboration in genomic prediction and genome-wide association without sharing data. Specifically, he will present on meta-analysis, transfer learning and federated learning in the context of genomic best unbiased linear prediction models.
Presenters

Hao Cheng is an assistant professor of quantitative genetics in the Department of Animal Science at the University of California Davis. His research interests are broadly involved in the development of statistical and computational methods for the (genetic) improvement of populations through more accurate, efficient, and biologically meaningful analysis (learn more at https://qtl.rocks).
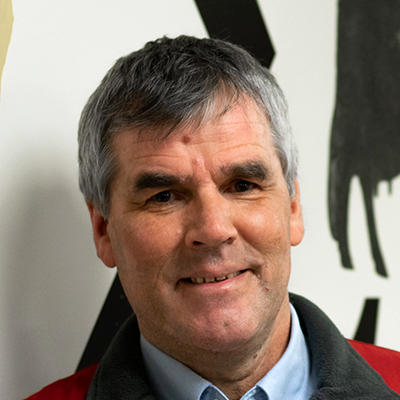
Jack C. M. Dekkers is a C.F. Curtiss Distinguished Professor in the Animal Science Department at Iowa State University. He grew up in the Netherlands and earned B.Sc. and M.Sc. degrees from Wageningen Agricultural University, and a Ph.D. in animal breeding from the University of Wisconsin. The focus of his research is on the integration of genomics and quantitative genetics to discover the genetic basis and enhance genetic improvement of traits of importance in livestock
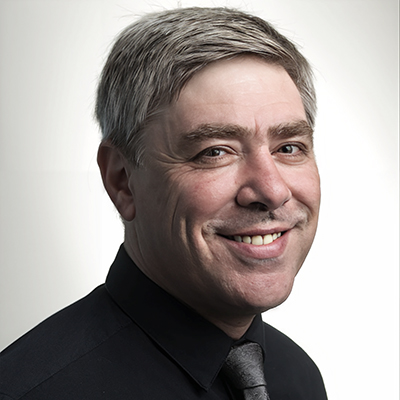
Juan P. Steibel is a Professor of Animal Science and J. Lush Endowed Chair of Animal Breeding and Genetics at Iowa State University. He obtained his B.S. in Agronomy from National University of La Pampa - Argentina, an M.S. in Biometry from University of Buenos Aires - Argentina, and a Ph.D. in Animal Science from Michigan State University. He investigates the development, adaptation, and application of statistical and computational methods to advance animal breeding and animal production through the integration of multi-omics data streams.